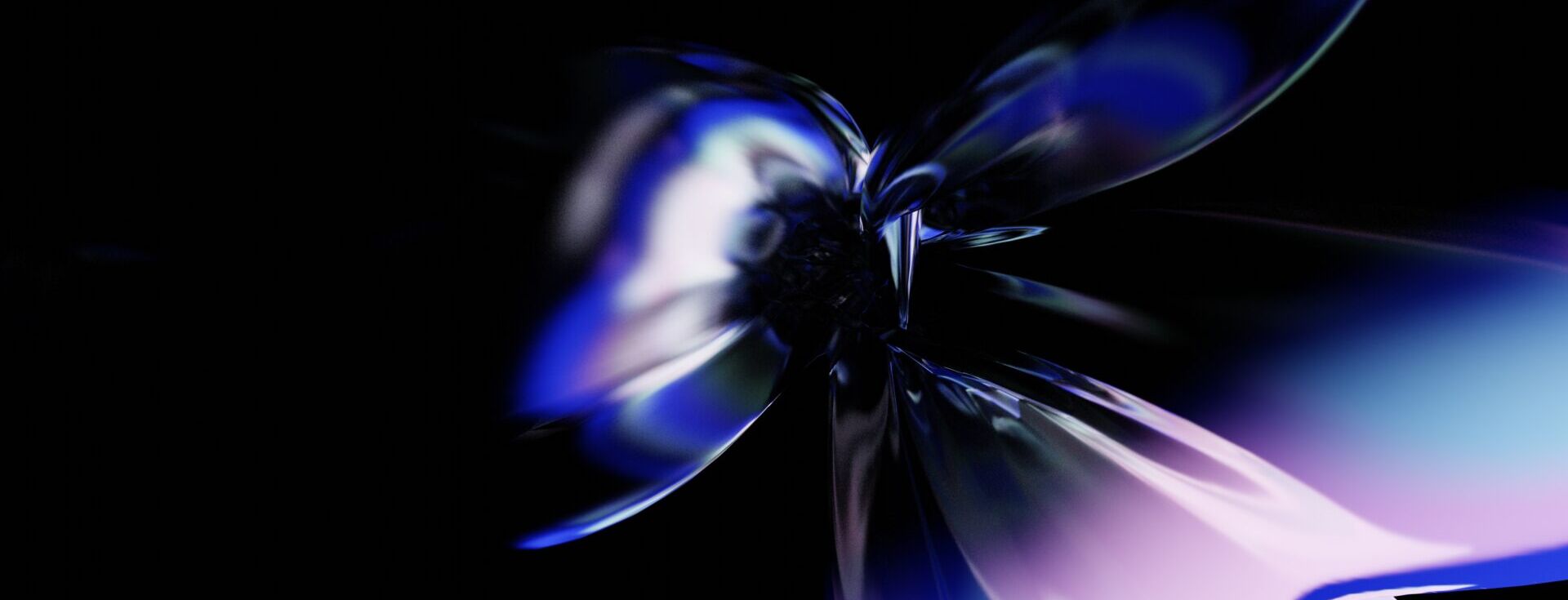
- Home
- Digital transformation
- How 4chan and The_Donald Influence the Fake News Ecosystem (By Jeremy Blackburn, University of Alabama at Birmingham)
How 4chan and The_Donald Influence the Fake News Ecosystem (By Jeremy Blackburn, University of Alabama at Birmingham)
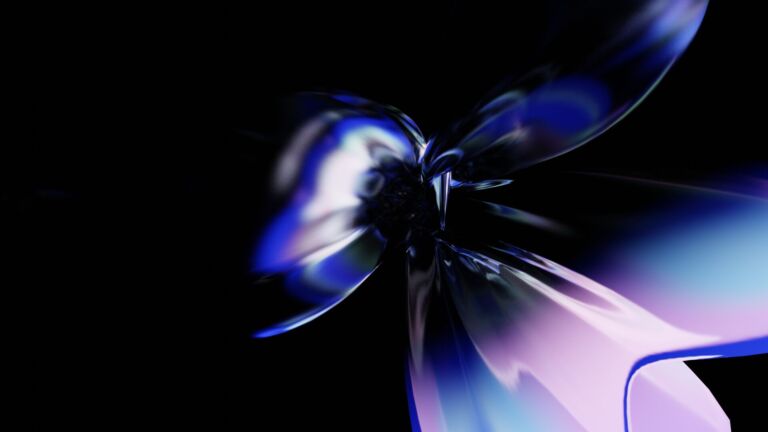
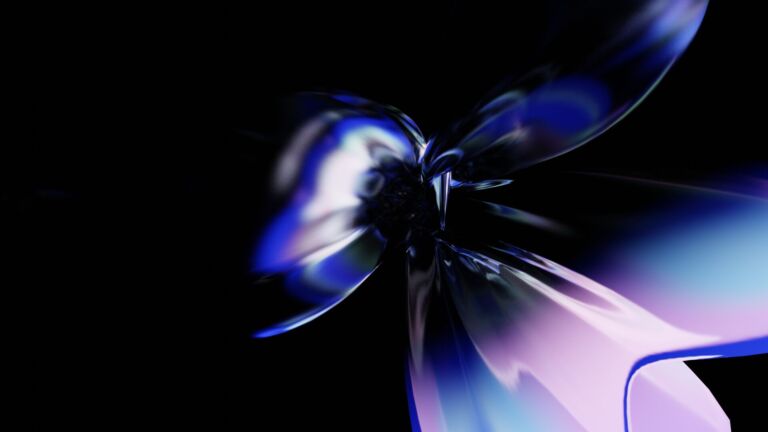
On July 2nd, 2017, Donald Trump, the President of the United States of America, tweeted a short video clip of himself ‘punching out’ a CNN logo. The video was modified so it looked as if Mr Trump was at a Wrestlemania event. It originally appeared on Reddit’s /r/The_Donald subreddit. Although The_Donald was already infamous in some circles, the uproar the image caused was for many the first introduction to a community of users that have had a striking amount of influence on the world stage. While memes like the one birthed from The_Donald are worrying, but mostly harmless, a shocking amount of disinformation (‘fake news’) is also created by and spread by smaller, more fringe, Web communities that exert a relatively vast influence on the greater Web.
In a nutshell, the explosion of the Web has commoditized the creation of false information and enabled it to spread like wildfire on an unprecedented scale. After a decade and a half of experience with social media platforms, bad actors have honed their techniques and been surprisingly adept at crafting messages that at best make it difficult to distinguish between fact and fiction, and at worst propagate dangerous falsehoods.
While recent discourse has tried to blur the lines between real and fake news, there are some fundamental differences. For example, the simple fact that fake news has to be created in the first place. Real news, even opinion pieces, are based around reporting and the interpretation of factual material. Not to dismiss the efforts of journalists, but, the fact remains: they are not responsible for generating stories from whole cloth. This is not the case with the type of misinformation pushed by certain corners of the Web.
Consider recent events like the death of Heather Heyer during the Charlottesville protests earlier this year. While facts had to be discovered, they were facts, supported by evidence gathered by trained professionals (both law enforcement and journalists) over a period of time. This is real news. However, the facts did not line up with the far-right political ideology espoused on 4chan’s /pol/ board (or if we want to play Devil’s Advocate, it made for good trolling material), and thus its users set about creating alternative narratives. Immediately they began working towards a shocking, to the uninitiated, nearly singular goal: deflect in any possible way from the fact that a like-minded individual committed a heinous act of violence.
4chan: Crowdsourced Opposition Intelligence
With over a year of observing, measuring, and trying to understand the rise of the alt-right’s online activities, we saw a familiar pattern emerge: crowdsourced opposition intelligence.
/pol/ users mobilized in a perverse, yet fascinating, use of the Web. Dozens of, and often conflicting, discussion threads putting forth alternative theories of Ms Heyer’s killing, supported by everything from pure conjecture to dubious analysis of mobile phone video and pictures, to impressive investigations discovering personal details and relationships of victims and bystanders. Over time, pieces of the fabrication were agreed upon and tweaked until it resembled in large part a plausible, albeit eyebrow-raising, false reality ready for consumption by the general public. Furthermore, as bits of the narrative are debunked, it continues to evolve, weeks after the actual facts have been established.
One month after Heather Heyer’s killing, users on /pol/ were still pushing fabricated alternative narratives of events.
The Web Centipede
There are many anecdotal examples of smaller communities on the Web bubbling up and influencing the rest of the Web, but the plural of anecdote is not data. The research community has studied information diffusion on specific social media platforms like Facebook and Twitter, and indeed each of these platforms is under fire from government investigations in the US, UK, and EU, but the Web is much bigger than just Facebook and Twitter. There are other forces at play, where false information is incubated and crafted for maximum impact before it reaches a mainstream audience. Thus, we set out to measure just how this influence flows in a systematic and methodological manner, analyzing how URLs from 45 mainstream and 54 alternative news sources are shared across 8 months of Reddit, 4chan, and Twitter posts.
While we had many interesting findings, there are a few we will highlight here:
- Reddit and 4chan post mainstream news URLs at over twice the rate than Twitter does, and 4chan in particular posts alternative news URLs at twice the rate of Twitter and Reddit.
- We found that alternative news URLs spread much faster than mainstream URLs, perhaps an artifact of automated bots.
- While 4chan was usually the slowest to a post a given URL, it was also the most successful at ‘reviving’ old stories: if a URL was re-posted after a long period of time, it probably showed up on 4chan originally.
Graph representation of news ecosystem for mainstream news domains (left) and alternative news domains (right). We create two directed graphs, one for each type of news, where the nodes represent alternative or mainstream domains, as well as the three platforms, and the edges are the sequences that consider only the first-hop of the platforms. For example, if a breitbart.com URL appears first on Twitter and later on the six selected subreddits, we add an edge from breitbart.com to Twitter, and from Twitter to the six selected subreddits. We also add weights on these edges based on the number of such unique URLs. Edges are colored the same as their source node.
Measuring Influence Through the Lens of Mainstream and Alternative News
While comparative analysis of news URL posting behaviour provides insights into how Web communities connect together like a centipede through which information flows, it is not sufficiently powerful to quantify the specific levels of influence they have.
To address this, we used a cool statistical model known as Hawkes processes. There’s a more technical treatment in our paper, but imagine users on Reddit, 4chan, and Twitter as part of systems that are stimulated and respond by posting URLs. There are a variety of stimuli these systems respond to. From organic discovery of URLs via surfing, to seeing a friend share it on Facebook. Sometimes these systems influence each other, for example, Donald Trump posting a video from Reddit’s The_Donald on Twitter. Hawkes processes let us quantify the stimulus-response relationship between different systems, and furthermore, accounts for the influence of other systems that we do not measure, and may not even know about!
An example of what a sequence of events on a Hawkes model with three processes might look like, using The_Donald, Twitter, and /pol/ communities for representative purposes. First, an event 1 (a URL being posted) occurs on Twitter; this is caused by the background rate of the process, meaning that the URL was posted not because it was seen on any of the communities in the model, but because it was seen elsewhere (including a user finding it organically). This initial event causes an impulse response on the rates of the other processes, The_Donald and /pol/, meaning that the URL is more likely to be posted on those platforms after having been seen on Twitter. Eventually, this causes another event on The_Donald (2), which in turn causes an event on /pol/. A process can cause an additional impulse response to itself, as seen with event 3, and multiple events can be caused in response to a single event, as seen with event 4 causing events 5.1, 5.2, and 5.3. Naturally, the data we collect does not explicitly state which events are caused by other events, or which are caused by the background rate.
Using Hawkes processes, we measured the influence of Reddit (more specifically, six subreddits with a substantial amount of news URLs: The_Donald, worldnews, politics, news, conspiracy, and AskReddit), 4chan, and Twitter on each other. We found that Twitter does have heavy influence on the spread of fake news, confirming concerns of other researchers and lending credence to government investigations. However, it does not exist in a vacuum, and is subject to the influence of other, lesser known ‘fringe’ communities. More specifically, The_Donald and /pol/ are responsible for around 6% of mainstream news URLs over 4.5% of alternative news URLs on Twitter. Keeping in mind the relative size of the communities (Twitter is several orders of magnitude bigger than 4chan or any particular subreddit), these findings are quite striking.
Hawke’s processes allow us to quantify the percentage of news URLs appearing in one community that are caused by another. This table shows the estimated mean percentage of alternative URL events on a community in a given column caused by alternative news URL events from a community in a given row (A), the estimated mean percentage of mainstream news URL events caused by mainstream news URL events (M), and the difference between alternative and mainstream news (also indicated by the color).
Moving Forward
Our work is a first step toward rigorously measuring the spread of fake news and the influence of social media, but much is left to be done. We now know that these dangerous fringe communities are not confined and we cannot study them in isolation, for example, just recently misinformation from 4chan related to the Las Vegas shooting spread across the Web. Considering the increasing awareness of the impact fake news has had in influencing referendums, elections, and public opinion in general, we expect an increase in funding for research trying to address it. To that end, we believe there are several specific areas that should be looked into. Machine learning models to automatically gauge the veracity of content should take into account its source and propagation path. This, of course, requires discovery and monitoring of multiple communities on the Web, which comes with a host of additional challenges. Finally, we think the research community should continue to build up our understanding of how this content is created; a deep enough understanding could allow us to adapt the strategies of bad actors as a tool against them. It’s time to fight fire with fire.
Jeremy Blackburn is an Assistant Professor in the Computer Science department at the University of Alabama at Birmingham. His work has covered a variety of topics ranging from cheating in online video games to cryptographic communication protocols for the Web. Before joining UAB, Jeremy was an associate researcher at Telefonica Research in Barcelona, Spain.
This article originally appeared on Bentham’s Gaze.
the newsletter
the newsletter