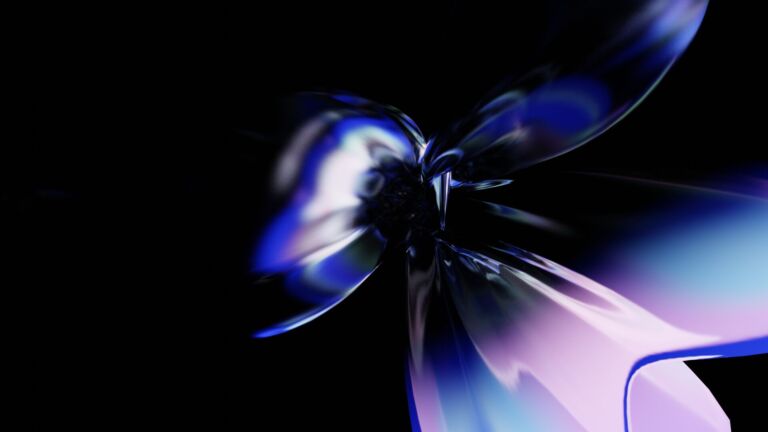
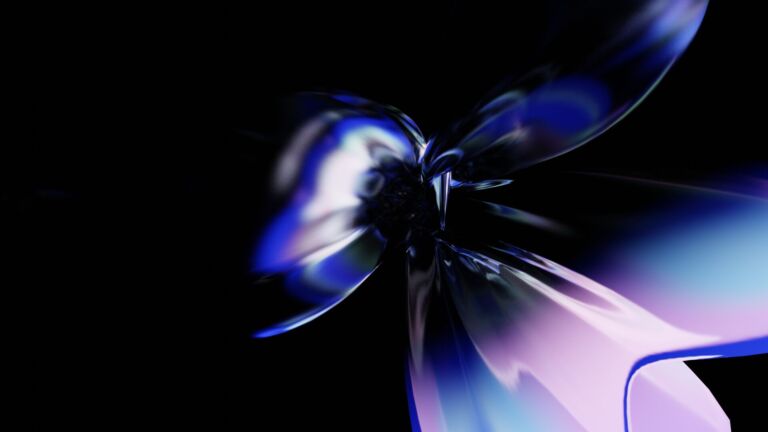
Introduction
It has always been shown that attackers innovate faster than defence teams. According to the report in M-Trends 2016, innovation allows the hackers to have an operating window of 143 days on average before being detected with conventional solutions and traditional security infrastructure.
This observation has incited numerous companies to turn to new methods and technologies to reduce as far as possible the window of attack. In this article we will be looking at 3 new techniques to detect and respond to cyber attacks, with practical implementations and feedback.
Threat Intelligence
Companies have thus invested considerably and for a very long time, in order to get as much information as possible, from classical security solutions like SIEM (Security Information and Event Management), IPS/IDS (Intrusion Prevention and Intrusion Detection Systems) and infrastructure solutions (firewall, proxy, etc.). As a result, they receive on average 17,000 alerts per week concerning malware, according to the Ponemon Institute. However, in actual fact, only 4% of these alerts are really investigated. The main reason being that the relevant signs of an intrusion are simply drowned out in a mass of data, interference and white noise. To solve this problem, the security teams need to configure and fine-tune their solutions and systems more effectively, in order to produce fewer alerts.
Thus, the objective of a Cyber Threat Intelligence (CTI) platform is to significantly reduce the time spent by data analysts consolidating and rationalising the threats they receive. This service will provide security experts with information on trends on current attacks, collect and seek important indicators of compromise (IOCs) like malicious IP addresses, URLs of attack, hashes/fingerprints of malicious files, etc.
This service will also enable more accurate identification of:
* the sources of threats and useful data in the corporate environment
* the motivation of the operator of the threat (i.e. the attacker)
* the risks the company is running following data breach, such as financial losses or damaged reputation
* the attacks other companies have fallen victim to
* the type of attacks with which the company is likely to be confronted, depending on priorities.
The IOCs and information collected can be used proactively by elaborating correlation or filtering rules between security components (firewall, IDS/IPS, Anti-DDoS), as well as during the investigative and response to incident stages.
Nevertheless, CTI cannot be the only major method of defence. In order to fight modern threats, it is vital to have a tool box with different defensive measures.
Automation of the response to incidents
With the right people and the right tools, CTI acts as a lever for the teams using it, allowing them to use the other tools and security measures more effectively. The security teams can thus concentrate on what is important: the most critical elements and attacks.
However, it is not unusual today to see CSIRT or SOC teams dealing with hundreds of security incidents every day at the risk of not properly describing or identifying the most important attacks. Add to that the heavy workload associated with every incident reported – collecting data, creating incident tickets, sending emails, generating reports, etc. – you can quickly measure the two-fold punishment suffered by the incident response team.
Bearing this observation in mind, it has become indispensable to build intelligent and automatic responses to common threats, which are either known or with mature feedback. This will reduce the response time from several days to a few minutes and enrich the knowledge base of internal and external threats.
Let’s take an example to illustrate the new potential methods.
When an alert is generated by the security solutions (IDS/IPS, SIEM, proxy), the cyber analyst of the SOC/CSIRT must understand quickly what is happening and how to contain an immediate threat, but that can take hours. When terminals are involved, the analyst might have to start a remote session, communicate with the user, conduct in-depth analyses of a suspected attack, etc.
With an optimised approach, when an attack is generated or an IOC feed is received, they are forwarded to an EDR function (Endpoint Detection and Response), which is basically a stealth agent working on the machines. It will launch an automatic search on new files, event logs and registry keys, collect the evidence, dump the RAM, and even stop suspicious processes. In the best case scenario, this stage would take several hours (5 or 6) when dealt with manually, or a few minutes at the most with an appropriate tool.
Thus, by automating the investigation and consolidation of events, the automated response tools enable to quickly determine if the alerts are benign or real threats, and in the latter case, to contribute to containing the threat as quickly as possible.
However, a major disadvantage of this approach is that false positives have a real impact on production. Indeed, despite being mere interference, they are likely to trigger automatic remedial actions. It is also important to note that the repetition of automated actions will reduce the competencies and knowledge of the SOC/CSIRT teams who will no longer receive feedback and get to know the origin and sequence of events and will only have a fragmented picture of the symptom (small scale) without knowing the cause or apprehending the attack in its entirety (large scale).
Machine Learning
Another possible function brought by these agents is the creation of a base of normal or common behaviours of a user or a system in the form of a snapshot. This base will then be used to detect any abnormal activity outside the zero line/baseline (processes, registry keys, files, connection or activity).
Currently, the “machine learning” phenomenon is transforming an increasing number of industries and has become a buzzword in numerous technological companies. At a time when more and more jobs are being taken over by robots and artificial intelligence, would it be conceivable to delegate the complex responsibility of cybersecurity to machines? The topic is already very controversial!
The current implementations enable the system to collect data by combining an agent working on client stations and sensors placed in segments of the network. The data is sent to intelligence engines analysing behaviour that use automatic learning to classify the samples collected to detect normal behaviour and anomalies.
This self-learning approach uses continuous monitoring and could resolve the issue of detection of new threats by creating a DNA of specific behaviour and by being independent of rules and signatures.
The main argument against these solutions is that they create too many false positives. Human intervention in weighting and validating the alerts during and after the learning phase compensate for this disadvantage. It would seem that the twosome man-machine/artificial intelligence will still remain the most effective solution against hackers and cybercriminals!
Conclusion
The world of cybersecurity is not entirely powerless or defenceless when it comes to hackers or cybercriminals, who are increasingly competent, determined and organised. We are presently experiencing rapid growth at the dawn of a new era that will provide us with more relevant tools and technologies. Unfortunately, we are still at the beginning of a long road that will force us to come back to the basics of “Security by Design” for all the components of the IS.
References
https://www2.fireeye.com/rs/848-DID-242/images/Mtrends2016.pdf
https://en.wikipedia.org/wiki/Secure_by_design
A Survey of Data Mining and Machine Learning Methods for Cyber Security Intrusion Detection, Anna L. Buczak and Erhan Guven, 2016
Artificial Intelligence in Cyber Defense, Enn Tyugu, 2011
About the author
Helmi RAIS is an expert in cybersecurity with about fifteen years of experience. He is currently a Senior Manager at Alliacom and Team Manager of AlliaCERT (Alliacom Computer Emergency Response Team). Helmi RAIS is a speaker and panel member at about fifty events related to cybersecurity (TEDx, ITU, FIRST, OIC-CERT, TFCSIRT, CCDF, CNIS Mag, Securiday). He is a founder member of several entities (ANSI, AlliaCERT, TUNCERT, OIC-CERT, AfricaCERT DevTeam) and is a member of the French ISC2 Chapter.
the newsletter
the newsletter